Monica Schoonhoven, Global Director of Customer Success at Textkernel, explains in this blog post which things you need to consider while identifying transparency in regards to AI.
Looking at how Large Language Models (LLMs), a form of generative AI capable of understanding and generating text (such as ChatGPT), are developed, it is, as with other AI models, still with data with which they are trained.
Each model is trained with a set of data. And the training set for LLMs is larger than ever. This is partly because data processing is faster and there is much more computing power. Whereas five years ago a model was trained on a few hundred to thousands of documents, LLMs today are trained on a large portion of the entire Internet, meaning they have thus been able to learn from much more data. However, it is important to realize that there is always human input when developing LLMs.
TRANSPARENCY IN AI: WHERE WILL THIS END?
With advances in technology and increased processing speed, we are seeing the reasoning of LLMs, relative to traditional AI, become much more complex, Monica explains. “LLMs are not necessarily complex, but they have much more depth. For example, they can better interpret what a particular question means or they can combine multiple sources simultaneously into a clear answer.”
In addition, the output that LLMs provide has increasingly come to resemble a human form of communication. Monica: “Think about putting a certain communication structure, friendliness and manners in different languages.” Those elements mean that the input, the processing of that input as well as the output are more sophisticated than ever. “And with that, you also see that the concern about a number of facets – where does something come from, why is a certain answer given, is the answer from ‘man’ or ‘a computer’ – is less and less clear.”
Monica continues: “So the key question is really, ‘Where will this end?’ Transparency is already somewhat in question, and with irresponsible use of AI, transparency could be lost completely.”
The GDPR focuses on what data you are allowed to use, the EU AI Act is about how you use this data
MONICA SCHOONHOVEN, GLOBAL DIRECTOR OF CUSTOMER SUCCESS
EU AI ACT TO ENSURE TRANSPARENCY
To ensure transparency in AI and that AI cannot harm society, the EU AI Act was created. In Europe, the main goal is to promote trust in AI technology. But in addition, it also aims to protect citizens from the impact AI might have. The passing of the EU AI Act happened recently, so not everything is yet known about all the specific implications and the exact implementation of the law.
Monica: “If we look at EU AI Act, it is very likely that the processes regarding recruitment will be classified as High Risk AI. The rules that will apply to this are in the following areas: transparency and accuracy, risk analysis, log keeping, human oversight and right to complaint and explanation.“
The European Parliament considers it incredibly important that companies, working with AI, provide insight and transparency, in order to ensure that citizens are permanently protected.
AI TRANSPARENCY IN PRACTICE
The main question then is how to apply this in practice. How can you check whether the AI technology you use or want to use actually complies with the EU AI Act. “The exact ground rules are not yet known,” Monica explains. “But there are already three very clear items you can raise with your AI technology provider around identifying transparency. Textkernel started the concept of ‘Responsible AI’ years ago, because not only is it important that we comply with (future) regulations, but we are also intrinsically motivated to stay very keen on transparency in recruitment processes, while also continuing to innovate.” Below, Monica elaborates on things to consider while identifying transparency in regards to AI: the data, the process and the monitoring.
THE DATA
What data is used? As mentioned, every AI model is trained on data. And since we’re talking about candidates and their information during recruitment processes, it’s important to look at the GDPR here as well. Because where the GDPR focuses on what data you are allowed to use, the EU AI Act is about how you use this data.
In addition, the accuracy of that data is very important. Does the data you feed the system to learn actually fit the task for the output. Verification of data is incredibly important at this point. Is it in the right language, is the data “clean” and appropriate for the intended application? Then again, more data is not necessarily better. For example, it’s better to have a smaller set with just the right inputs so that the result will also be more accurate. So sometimes LLMs trained on the ‘Internet’ actually give less good results than ‘Not-So-Large Language Models’ , due to, for example, semi-manually controlled, smaller volume input data sets.
Representativeness is also an important element, because at this point is also the major concern regarding bias (or bias). It is important to ensure that training data is a diverse and balanced representation of the population. If it is not, certain groups may be disadvantaged by the algorithm. Make sure you know where the data is coming from. One element that helps with this, and we do at Textkernel, is to create an AI Fairness Checklist. With that, you create certain standards that you can hold yourself to. That way you have quicker insight into where the data is coming from and what kind of data is in your training set. You can then also immediately make sure that any input bias disappears.
As a final element, updates are very important. How do you ensure continuous compliance with the latest rules and what processes have been agreed upon for this purpose? An AI model is as old as the data put into it. For some processes, a year old data is actually too old, think here for example of job and skills developments.
The aforementioned AI Fairness checklist is part of a larger Risk Management Framework (RMF) within Textkernel, which is now being implemented in the context of the EU AI Act. The RMF is designed to identify, measure and capture risks wherever possible. By creating the AI Fairness checklist, Textkernel is at the forefront of this by ensuring that our clients are always well within standards.
THE PROCESS
One of the biggest pitfalls in automating a series of multiple tasks is the snowball effect. By this we mean that the more steps of the entire recruitment process you automate in succession, the more the loss of transparency “piles up. Be careful not to let AI take over the entire process from start to finish, because a piece of transparency and accuracy disappears. Textkernel recommends making sure that during each step in the application process the AI model is trained task-by-task, so that each step in the process does not suffer from the snowball effect. That way, you prevent erroneous output without human oversight from affecting the steps that come after, and ensure that accuracy and transparency actually improve. After all, you provide an AI model trained on a specific task with a focus on accuracy to the training data.
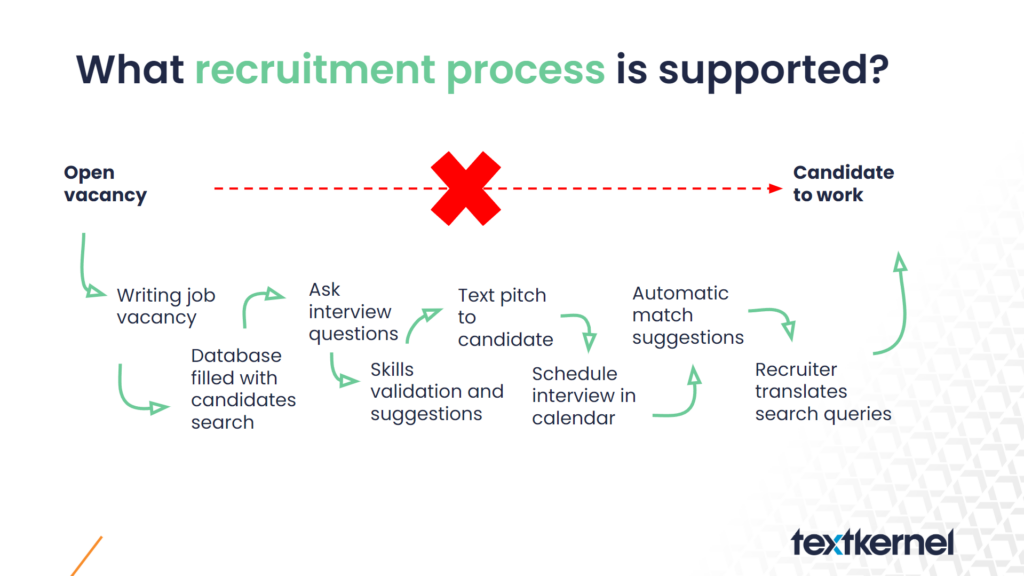
THE MONITORING
Ultimately, you’re looking for a solution where the recruiter is always in control. That means, on the one hand, that you have to provide insight into how the results came about and, on the other hand, that you have to be able to adjust the results, remove them completely or take over manually yourself. This is also called a white box solution.
To ensure transparency around AI in your organization, it is important to know how your data is being used. Also, the accuracy of the data is incredibly important. But note here: more data does not always mean it is actually better. Third, it is important to ensure that your data is a diverse and balanced representation of the population. Finally, you need to make sure that you always stay compliant with the latest rules and processes.
RESPONSIBLE AI
Using AI can be a double-edged sword. It can cause harm when used carelessly, but it can equally promote fairness and reduce bias when employed responsibly – which is crucial to ensuring ethical outcomes. At Textkernel, we are dedicated to championing Responsible AI in Recruitment, placing a premium on ethical practices and inclusivity to pave the way for a brighter, more equitable future.
Learn more on our Responsible AI page.